Lead Generation Drug Discovery
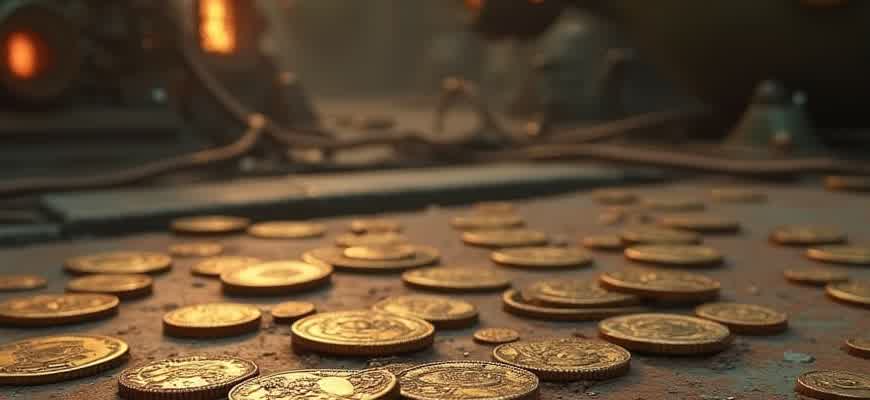
The intersection of blockchain technology and pharmaceutical research is creating new opportunities for the discovery of novel drugs. Traditional drug development is a lengthy and expensive process, often taking years before a new medication reaches the market. By integrating cryptocurrency and decentralized platforms, pharmaceutical companies can expedite this process, reduce costs, and increase transparency throughout the research phase.
One of the most significant innovations is the ability to tokenize the drug discovery process. Blockchain enables secure and transparent tracking of research data, intellectual property, and funding allocation. This ensures that each step of the process is verifiable and accountable, which is essential for building trust among investors and stakeholders.
- Data Security: Ensures protection from data tampering, a common issue in traditional research environments.
- Decentralization: Promotes collaboration among research institutions and startups without intermediaries.
- Cost Efficiency: Reduces transaction fees and administrative costs through the use of cryptocurrencies.
Additionally, blockchain-based platforms facilitate the creation of decentralized autonomous organizations (DAOs) that allow for community-driven funding and decision-making. This model provides a direct channel for investors to participate in the development of pharmaceutical innovations.
"By utilizing decentralized technologies, pharmaceutical companies can not only accelerate the research timeline but also ensure a fair distribution of rewards among all participants." - Industry Expert
Benefits | Impact |
---|---|
Secure Data Storage | Prevents data breaches and fraud, ensuring reliability in research findings. |
Decentralized Funding | Enables global investors to contribute to drug development, reducing reliance on traditional financial institutions. |
Blockchain's Impact on the Process of Identifying Potential Drug Candidates
Blockchain technology, renowned for its decentralized nature and high level of security, is now beginning to play a significant role in the pharmaceutical sector. Specifically, it is transforming how companies approach the initial stages of drug development, known as lead identification. By using blockchain, researchers can securely share data, manage intellectual property, and track experimental results with greater transparency and trust. This could lead to faster identification of viable compounds while reducing the risk of fraud and data manipulation, which has historically been a significant challenge in the field.
Furthermore, blockchain's ability to streamline the data-sharing process makes it easier for pharmaceutical companies to collaborate across borders. As the pharmaceutical industry becomes increasingly globalized, having a secure, transparent, and accessible system for storing and exchanging drug discovery data is crucial. This technology not only increases efficiency but also enhances the traceability of drug development efforts, ultimately contributing to more effective and accelerated lead generation.
Key Benefits of Blockchain in Lead Identification for Drug Discovery
- Enhanced Data Integrity: Blockchain ensures that all data related to drug discovery is immutable and securely stored, reducing the chances of tampering or data loss.
- Improved Collaboration: Researchers from different institutions can share their findings in real-time, helping speed up the discovery of promising drug candidates.
- Smart Contracts for Licensing: Blockchain can automate the licensing of intellectual property (IP) associated with drug candidates, ensuring fair and timely payments for all parties involved.
- Increased Transparency: Blockchain’s decentralized ledger ensures that all data is available to authorized parties, improving the transparency of drug discovery processes.
"Blockchain has the potential to transform the way we generate leads in drug discovery, enabling more effective collaborations, reducing data fraud, and enhancing overall transparency."
Blockchain-Enabled Drug Discovery Pipeline
Stage | Blockchain Contribution |
---|---|
Data Collection | Blockchain provides secure and verifiable data storage, ensuring that data is consistent and accessible for all collaborators. |
Lead Identification | Decentralized networks allow for faster sharing and validation of experimental results, helping identify potential leads more quickly. |
Intellectual Property Management | Smart contracts automate licensing agreements, ensuring secure and transparent management of intellectual property rights. |
Optimizing Compound Identification Using AI and Machine Learning in Drug Discovery
The pharmaceutical industry has seen significant advancements in drug development through the application of artificial intelligence (AI) and machine learning (ML). These technologies are increasingly being used to streamline the identification of lead compounds, reducing the time and cost associated with traditional discovery methods. By leveraging data-driven approaches, AI can efficiently analyze vast chemical libraries and predict potential drug-like properties, enhancing the selection process for early-stage drug candidates.
In particular, AI and ML algorithms can sift through large datasets of molecular structures to identify patterns that are often too complex for human researchers to detect. By integrating these tools into the early phases of drug discovery, researchers can significantly improve the accuracy of lead identification, minimizing trial-and-error methods. These innovations are crucial in accelerating the path to viable drug candidates and, ultimately, market-ready medications.
Key Benefits of AI and ML in Lead Generation
- Increased Efficiency: AI systems can analyze vast chemical libraries in a fraction of the time compared to manual methods.
- Enhanced Predictive Power: Machine learning models can predict how certain compounds will interact with biological targets, improving early-stage decision-making.
- Cost Reduction: By identifying promising candidates early on, AI reduces the need for expensive experimental testing and rework.
"AI and ML can uncover insights that traditional methods miss, leading to faster, more effective drug discovery processes."
Key Approaches to AI in Drug Discovery
- Data Mining: AI algorithms analyze historical data from previous drug trials, identifying correlations between compound structures and therapeutic efficacy.
- Predictive Modeling: Machine learning models are trained to predict molecular interactions, optimizing the design of compounds for specific biological targets.
- Virtual Screening: AI is used to conduct virtual simulations of compound interactions with proteins, helping prioritize compounds for experimental validation.
Applications of AI in Compound Screening
Method | Benefit | Example |
---|---|---|
Deep Learning | Improves compound selection by identifying complex patterns in molecular data. | Identifying new inhibitors for cancer treatment through neural networks. |
Generative Models | Generates novel compounds based on desired characteristics. | Designing new antibiotics to overcome resistance. |
Natural Language Processing | Extracts valuable insights from scientific literature and patents. | Identifying potential compounds for rare diseases by analyzing published research. |
Identifying Targeted Biomarkers for Accurate Lead Discovery
In the rapidly evolving field of drug discovery, identifying specific biomarkers plays a crucial role in developing targeted therapies. By pinpointing distinct biomarkers associated with disease pathways, researchers can better understand the molecular basis of conditions and select appropriate compounds to develop as potential drugs. These biomarkers, when accurately identified, provide a foundation for effective lead discovery, enabling a more efficient and precise therapeutic development process.
However, the complexity of biomarker identification requires the integration of advanced technologies such as genomics, proteomics, and high-throughput screening methods. This integration allows for the identification of novel targets that could otherwise remain undetected, particularly in diseases with complex pathophysiology. In this context, cryptographic technologies and blockchain applications in drug discovery are being explored for their potential to securely manage large datasets, ensuring data integrity and improving the reproducibility of results.
Key Approaches to Target Biomarker Discovery
- Genomic Analysis: Leveraging next-generation sequencing (NGS) to uncover genetic mutations and alterations in disease-specific genes.
- Proteomic Profiling: Employing mass spectrometry and antibody-based techniques to identify proteins that act as biomarkers for disease progression.
- Machine Learning Algorithms: Applying AI-driven approaches to analyze vast datasets for the discovery of novel biomarkers.
Once biomarkers are identified, a series of validation steps are required to confirm their relevance in drug discovery:
- Validation in Preclinical Models: Testing the biomarkers in animal models to ensure their role in disease progression.
- Clinical Validation: Conducting clinical trials to validate the biomarkers' effectiveness in human subjects.
- Integration with Therapeutic Development: Ensuring the biomarkers align with existing treatment pathways and can aid in identifying potential drug candidates.
“The integration of precise biomarkers into the lead discovery process is essential for identifying compounds with high therapeutic potential and minimal side effects. Blockchain and cryptographic solutions can secure data and ensure the authenticity of research findings.”
Challenges in Biomarker Discovery
Challenge | Potential Solutions |
---|---|
Data Overload | Utilizing blockchain to ensure secure storage and efficient retrieval of large datasets for analysis. |
Cross-Disease Relevance | Employing machine learning to identify biomarkers that are disease-specific, reducing false positives. |
Reproducibility | Leveraging cryptographic techniques to ensure the integrity and reproducibility of results in multiple research settings. |
How to Integrate High-Throughput Screening in Lead Generation
In the field of drug discovery, the ability to quickly identify potential drug candidates is crucial. High-throughput screening (HTS) has revolutionized this process by allowing researchers to test thousands of compounds in a short amount of time. Integrating HTS into the lead generation process can accelerate the identification of promising drug candidates, reducing both time and costs in the early stages of drug development.
By utilizing automated systems and advanced technology, HTS enables the rapid testing of chemical libraries against biological targets. The result is a more efficient and systematic approach to discovering leads that can be further optimized. The integration of HTS into lead generation requires careful planning, ensuring that the right assays, compounds, and technologies are selected to maximize its potential.
Steps for Successful HTS Integration in Lead Generation
- Selection of Assays: Choose assays that closely mimic the biological activity of the target. This step ensures that the compounds screened are relevant and have a high chance of being effective in vivo.
- Optimization of Compound Libraries: Curate a diverse compound library based on drug-like properties, such as solubility and permeability. A well-designed library increases the likelihood of finding a lead compound.
- Data Analysis: Employ advanced data analytics and machine learning to process large datasets. These tools help identify hits with high specificity and predict their pharmacological properties.
Key Insight: Integration of HTS requires not only advanced technology but also interdisciplinary collaboration, combining chemistry, biology, and data science to ensure that the results are actionable and lead to viable drug candidates.
Metrics for Evaluating HTS Success
Metric | Description | Target Value |
---|---|---|
Hit Rate | Percentage of compounds showing activity against the target | 10-15% |
Assay Quality (Z'-Factor) | Indicates the reliability of the screening assay | >0.5 |
Data Reproducibility | Consistency of results across multiple screening rounds | >90% |
Important Note: The success of HTS is not solely determined by the number of hits, but by the quality of the data and the predictive value of the lead candidates.
Evaluating Lead Compounds: Key Metrics and Validation Techniques
When identifying potential drug candidates, especially in the realm of blockchain-based research or cryptocurrency-backed biotech projects, it is crucial to assess lead compounds through various evaluation methods. These metrics are designed to predict how a compound will behave in biological systems and guide its progression from the discovery phase to preclinical and clinical studies. For blockchain-supported platforms, these validation steps may also be verified via decentralized protocols to ensure transparency and security in the process.
Key evaluation metrics for lead compounds include pharmacokinetic properties, toxicity profiles, and the compound's ability to bind to specific target proteins. Validation techniques, which often combine experimental methods with computational predictions, further refine these assessments. These steps can also be enhanced by integrating blockchain technology, ensuring that all data points are immutable and verifiable, thus increasing confidence in the compound's development trajectory.
Key Metrics for Lead Compound Evaluation
- Solubility: Determines how well a compound dissolves in biological fluids, influencing absorption and bioavailability.
- Target Binding Affinity: Measures how strongly a compound binds to its target, indicating its potential efficacy.
- Metabolic Stability: Assesses the compound's stability within the body and its resistance to metabolic breakdown.
- Toxicity Assessment: Evaluates the potential harm a compound may cause at therapeutic doses, ensuring safety in early clinical trials.
Validation Techniques for Lead Compounds
- In vitro Screening: Testing compounds in cell cultures to measure efficacy and potential toxicity before animal studies.
- In vivo Testing: Administering the compound in animal models to further assess pharmacokinetics and safety.
- Computational Modeling: Using machine learning and AI algorithms to predict the behavior of compounds based on prior data.
- Blockchain Validation: Implementing decentralized platforms to record experimental data, ensuring secure and transparent tracking of compound performance.
"By leveraging decentralized networks and AI-powered validation, biotech researchers can increase the credibility and efficiency of their lead compound development processes, bringing novel drugs to market faster and more securely."
Compound Evaluation and Validation Table
Metric | Evaluation Method | Outcome |
---|---|---|
Solubility | In vitro dissolution testing | Determines optimal dosing and delivery methods |
Target Binding Affinity | Surface Plasmon Resonance | Predicts compound efficacy |
Metabolic Stability | In vivo animal testing | Assesses drug longevity and bioavailability |
Toxicity | Acute toxicity tests | Ensures safety profile |
Building Strategic Partnerships for Scalable Lead Generation in Crypto
In the rapidly evolving cryptocurrency landscape, establishing effective partnerships is crucial for achieving scalable lead generation. The decentralized nature of blockchain and the intricacies of token economies require a robust network of collaborators to drive sustainable growth. Successful collaborations with blockchain platforms, influencers, and data-driven platforms enable a more extensive reach, which is essential in attracting and converting qualified leads into active users or investors.
Strategic partnerships in the cryptocurrency sector can offer significant advantages, such as improved market visibility, access to larger audiences, and enhanced credibility. These alliances can range from technical integrations with blockchain projects to joint marketing campaigns with crypto influencers. By aligning with organizations that share similar goals, companies can amplify their lead generation efforts and build a more effective and scalable pipeline.
Key Strategies for Building Effective Crypto Partnerships
- Identify Complementary Projects: Collaborate with projects that align with your target audience but offer different services or products. This creates opportunities for cross-promotion and joint marketing.
- Leverage Data Analytics: Use data-driven insights to identify potential partners with the highest conversion potential. This helps in selecting collaborations that will deliver measurable results.
- Token Incentives: Offer token rewards or stake incentives to users for participating in the partnership, creating a mutually beneficial environment that incentivizes both parties.
Benefits of Strategic Collaborations
Benefit | Impact on Lead Generation |
---|---|
Increased Brand Visibility | Expands reach and attracts new leads from partner networks. |
Enhanced Credibility | Trust is built through association with reputable projects. |
Access to Targeted Audiences | Tailored access to highly specific crypto communities. |
"A well-executed partnership within the crypto ecosystem can not only boost lead generation but also foster long-term growth by aligning incentives and leveraging shared networks."
Cost-Effective Methods to Improve Lead Discovery Pipelines
Drug discovery is an inherently expensive process, often requiring significant investment to generate and test potential drug candidates. In the quest to streamline and economize these pipelines, blockchain and cryptocurrency technologies offer promising cost-saving solutions. By enabling decentralized data exchange and reducing reliance on traditional intermediaries, these innovations can decrease both operational costs and the time needed to advance drug candidates through early-stage discovery.
Cryptocurrency can also provide an efficient method for compensating contributors in drug discovery projects, incentivizing global collaboration while minimizing transaction costs. By using microtransactions powered by cryptocurrency, pharmaceutical companies can make quicker, cheaper payments to researchers, facilitating more dynamic and responsive lead generation workflows. These methods can significantly reduce the financial barriers to participation and enhance the efficiency of the entire discovery process.
Practical Benefits of Cryptocurrency in Lead Discovery
- Blockchain for Efficient Data Management: Blockchain technology allows for secure, transparent data sharing without intermediary costs, ensuring greater reliability and reducing the risk of data duplication.
- Smart Contracts for Streamlined Collaboration: Automated agreements executed via smart contracts ensure faster, low-cost transactions while guaranteeing that all parties are fairly compensated based on agreed-upon milestones.
- Crypto-Based Rewards for Global Participation: Cryptocurrency can be used to reward global researchers, incentivizing data contributions and enhancing the speed of lead discovery by making transactions seamless and low-cost.
Cost-Reduction Impact of Blockchain and Cryptocurrency
Technology | Benefit | Cost Impact |
---|---|---|
Blockchain | Secure and transparent data sharing | Lower administrative costs, improved accuracy |
Smart Contracts | Automated contract execution | Reduced transaction fees, faster agreements |
Cryptocurrency Payments | Quick and inexpensive cross-border payments | Minimized overhead, enhanced global collaboration |
"By integrating blockchain and cryptocurrency into lead discovery pipelines, pharmaceutical companies can achieve both financial and operational efficiencies that transform the drug development landscape."
Overcoming Challenges in Lead Optimization During Early Drug Development
In the early stages of drug discovery, lead optimization plays a critical role in identifying potential drug candidates. However, this phase is fraught with challenges that can significantly impact the success of drug development. The optimization of lead compounds requires a deep understanding of their pharmacokinetic properties, toxicity profiles, and the ability to fine-tune their chemical structure to improve efficacy and minimize side effects. Failure to adequately address these challenges can lead to the rejection of promising candidates, increasing both time and cost for pharmaceutical companies.
One of the most significant hurdles in lead optimization is balancing potency with selectivity. While improving the potency of a drug is essential, it often comes at the expense of selectivity, leading to off-target effects. Additionally, maintaining a compound's stability while optimizing its pharmacological profile is a delicate task that requires precise molecular adjustments. Addressing these issues demands advanced techniques, such as high-throughput screening and computational chemistry, to predict interactions and identify the most promising candidates for further development.
Key Challenges in Lead Optimization
- Potency vs. Selectivity: Striking the right balance between effective drug action and avoiding unintended effects on non-target receptors.
- Drug Metabolism and Stability: Ensuring the compound remains stable and effective under physiological conditions.
- Toxicity Risk: Assessing and mitigating potential toxicological risks early in the development process.
- Formulation Challenges: Developing a suitable drug formulation for human administration while maintaining the compound’s bioavailability.
"The delicate balance between optimizing lead compounds and ensuring their safety and effectiveness remains one of the most complex aspects of early drug development."
Approaches to Overcome Optimization Challenges
- Computational Modeling: Using AI and machine learning to predict drug interactions, toxicity, and optimal structural modifications.
- Structure-Activity Relationship (SAR) Analysis: Systematically evaluating the impact of molecular modifications on biological activity.
- In Vitro and In Vivo Testing: Conducting a range of preclinical trials to assess the compound’s performance in biological systems.
Challenge | Approach | Outcome |
---|---|---|
Potency vs. Selectivity | Computational modeling and SAR analysis | Improved targeted potency with minimized off-target effects |
Drug Metabolism | In vivo testing and metabolic profiling | Enhanced drug stability and bioavailability |
Toxicity | Advanced toxicity screening technologies | Early detection of potential risks, reducing development time |